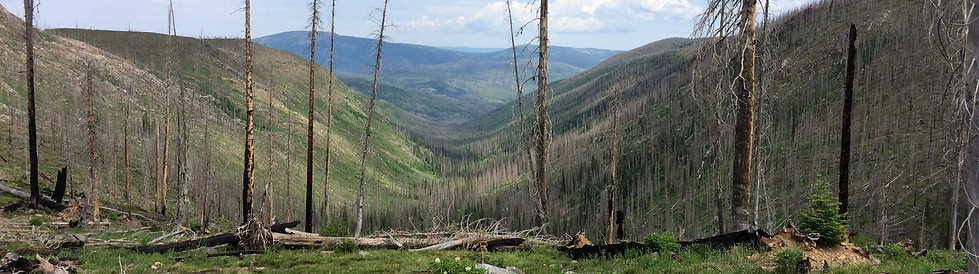
Regeneration following the Mt Zirkel Wildfire
In 2002, a large, severe wildfire burned vast expanses of Routt National Forest. The fire burned a great variety of forest stands in regards to species, age, and topographic position. Additionally, a large portion of the burned forest had been affected by a severe windstorm just five years prior. Over the past several years, we've been collecting data from permanent plots to observe how different parts of the forest are responding to this disturbance.
In some stands, we see abundant regeneration of one or more tree species. In others, no seedlings have been able to establish in the 13 years following the fire. It is our goal to determine which factors seem to be the most influential on the varying types and amounts of regeneration that we've observed. Which species are better able to regenerate in certain environments? How does postfire regeneration in stands that were blowndown in 1997 differ from regeneration in similar stands that were not blowndown? How influential are climatic factors, and how might a warming climate affect postfire regeneration in these types of forests? These are some of the many questions we are attempting to answer with this study.
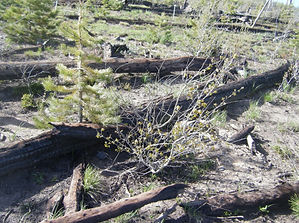
An important piece of this project is to model postfire regeneration throughout the burned area. With such a model, we would attempt to identify how the regeneration of each tree species is distributed across space and understand which factors (climatic, pre-fire forest characteristics, topographic, etc.) may be influencing the density of regeneration for each species. This model will also allow us to compare current forest dynamics with those that we may see in future years as climate continues to change.
The purpose of such a model is not to reveal exactly how many trees are regenerating, but rather to shed light on the mechanisms controlling regeneration. By predicting regeneration type and density with high accuracy, we can learn which predictor variables are most important.
Check the publications page for full text articles from this research.